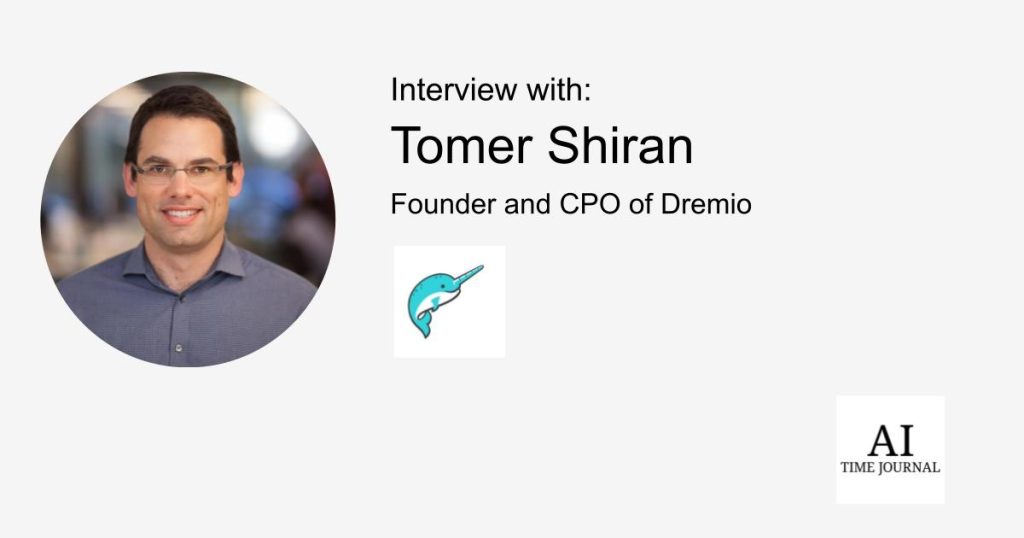
In this exclusive interview, we explore Tomer Shiran’s journey from shaping the Big Data movement at MapR to founding Dremio, a leader in the data lakehouse space. Tomer shares insights on innovations in open architecture and AI, strategies driving Dremio’s success, and his vision for the future of data analytics. Discover how Dremio is empowering businesses to unlock their full potential and redefine the way organizations harness their data.
You’ve been pivotal in shaping Dremio’s journey and its core technology since its inception. Can you share what inspired you to address the data lakehouse space specifically, and how that vision evolved?
When I founded Dremio, my inspiration came from a persistent frustration I observed—and experienced—while working with enterprise data systems. At MapR, I saw how complex and inefficient it was for companies to access, analyze, and gain value from their data. Organizations were spending so much time and money moving data between systems, locking themselves into proprietary platforms, and struggling to deliver insights quickly enough to keep pace with business needs. I wanted to solve these problems by creating a platform that combined the flexibility of data lakes with the high performance and ease of use traditionally associated with data warehouses.
I’ve always believed in the power of data to transform organizations, but that transformation is only possible when data is accessible and actionable. I envisioned a solution that would eliminate the barriers to working with data—removing reliance on traditional ETL processes, lowering costs, and enabling real-time insights directly from the source. This vision became the foundation for Dremio.
By building an open data lakehouse platform, we’ve made it possible for organizations to use their data without the heavy lift of moving it or dealing with vendor lock-in. Technologies like Apache Iceberg and Apache Arrow are critical to this mission, and I’m proud that Dremio has played a leading role in their development. These innovations reflect my commitment to empowering companies with the tools to unlock the full potential of their data, making analytics not just faster and easier, but more democratic and cost-effective.
At MapR, you played a crucial role as one of the early team members in the Big Data analytics movement. How did that experience influence your approach to leading Dremio and developing its core mission and values?
At MapR, my experience taught me how critical it is to create systems that are both robust and accessible to users of varying technical expertise. During my time there, I observed firsthand the challenges that large enterprises faced with early Hadoop deployments. While the technology held enormous potential, many companies lacked the engineering capacity to manage these complex systems effectively.
This understanding shaped my approach to product design and leadership at Dremio. For example, I saw the immense value in simplifying access to data while maintaining the performance and reliability needed at scale. Building solutions for enterprises highlighted the need for technologies that could bridge gaps in data interoperability while empowering non-technical users to derive insights easily. At MapR, this involved supporting customers as they struggled with siloed data and the complications of integrating different formats and tools—a challenge that strongly influenced Dremio’s mission to make data accessible and actionable without heavy IT involvement.
The idea of a data lakehouse optimizing both self-service analytics and AI is intriguing. Can you explain the technical and organizational challenges involved in building such a unified platform, and how you see Dremio’s approach standing out in this field?
Technically, the primary challenges include ensuring high-performance query execution, seamless integration with existing ecosystems, and managing governance across distributed architectures. Organizationally, it’s about driving alignment between data engineering and business teams. Dremio’s approach stands out with its open architecture—leveraging Apache Iceberg to ensure data freedom—and its focus on delivering self-service analytics without the curiosity tax of traditional cloud consumption models.
Dremio continues to strengthen its ongoing commitment to deliver open, scalable, and flexible lakehouse architectures that streamline data integration and analytics across any environment. As a result, our customers no longer have to choose between vendors or architectures as they can integrate with their preferred catalog, deploy on-prem, in the cloud, or in a hybrid architecture that ensures seamless interoperability across platforms, enabling unified analytics without being tied to a specific vendor.
Flexibility is critical for modern organizations looking to maximize the value of their data. Dremio empowers businesses to deploy their lakehouse architecture wherever it’s most effective and we remain 100% committed to giving customers the freedom to choose the best tools and infrastructure while reducing fears of vendor lock-in.
Generative AI is reshaping industries rapidly. From your perspective, how can organizations harness generative AI to transform data analysis workflows, and what new capabilities does it open up for business users?
Harnessing the power of generative AI to revolutionize data analysis workflows is an objective of most businesses today as they look to unlock the power of artificial intelligence for seamless data analysis. Generative AI can make this effort significantly more intuitive by enabling users to interact with data through natural language or auto-generated insights. For businesses, this unlocks opportunities to discover patterns and trends without deep technical expertise. It’s a game-changer for democratizing data access.
Our solution includes advanced AI-driven features that empower business users to query data with text, enhance data exploration, and accelerate insights. However that is only the beginning as we’re exploring additional ways to embed generative AI into workflows, enhancing user experiences and accelerating time to insights.
You’ve overseen Dremio’s growth from a small team to over 100 employees. What strategies have been most effective in maintaining innovation and agility as the team expanded, and how do you see this culture impacting Dremio’s future?
Fostering a culture of curiosity and collaboration has been key. We’ve focused on empowering teams to take ownership, encouraging cross-functional alignment, and maintaining a startup mentality even as we’ve scaled. This has allowed us to iterate quickly, stay customer-focused, and remain at the forefront of industry innovation.
“The driving force behind Dremio is always to do better. Clear communication, accountability, and respect are cornerstones for our employees. Our mascot “Gnarly the Narwhal” sets the standard for Dremio employees (a.k.a “Gnarlies”). We like approaching our jobs with a “gnarly” attitude that pushes us to achieve unprecedented outcomes”. Our Gnarlies are doing that daily. We also believe the workplace is where our Gnarlies can engage in a diversity of opinions yet come together on a common mission; enabling the next generation of data analytics.
Our core values form the foundation of how we collaborate as a team and may be one of the reasons Dremio was named one of the “2022 Best Places to Work in the Bay Area” by the San Francisco Business Times.
The ability for business users to query data in natural language represents a new frontier in data accessibility. What key technological breakthroughs make this possible, and what barriers remain in making text-based data queries universally reliable?
Advances in large language models (LLMs) and vector databases have made natural language processing (NLP) for data queries feasible. These technologies enable understanding of context and intent, making querying more intuitive. However, barriers include ensuring accuracy, handling ambiguous queries, and scaling to complex datasets. The challenge lies in refining these models to consistently deliver precise, actionable insights.
In your view, what role will automation play in enhancing data exploration and the speed of insights for companies? Are there specific automation-driven solutions within Dremio that you’re particularly excited about?
Automation will be pivotal in streamlining data preparation, enabling faster exploration, and identifying patterns that might otherwise go unnoticed. At Dremio, I’m excited about how our technology automates query optimization and integrates with open standards like Iceberg to reduce manual effort while delivering insights faster and more efficiently.
With your background in both engineering and product management, how do you approach balancing technical advancement with user-centered design, particularly when it comes to creating intuitive analytics tools?
It starts with understanding user needs deeply—listening to feedback and observing how our tools are used. Balancing technical innovation with simplicity is critical. At Dremio, our ongoing vision is to ensure that even our most advanced features are accessible and intuitive, empowering users without requiring them to be data experts.
Today’s technocentric business models demonstrate the need for a successful AI and analytics architecture. Simply put, making it easier for users is a table-stake and failure is not an option.
Looking at the future of data lakehouses, what emerging trends or technologies do you believe will be most transformative over the next five years, especially as they relate to scaling AI capabilities in businesses?
I see three transformative trends: the rise of AI-ready data, advancements in real-time analytics, and the growing adoption of open data architectures like Apache Iceberg. These trends will help businesses scale AI capabilities, reduce costs, and make data more actionable. Dremio is at the forefront of this evolution, building platforms that are both future-proof and flexible.
You’ve also founded two websites with a substantial user base. How has this experience influenced your approach to product development and customer engagement in enterprise technology, and are there any surprising similarities between building for consumers versus enterprises?
Building consumer websites taught me the importance of user-centric design and the power of a seamless experience. While enterprises have more complex needs, the underlying principles of simplicity, engagement, and responsiveness remain the same. In both domains, success hinges on solving real problems effectively and ensuring a strong connection with your audience.