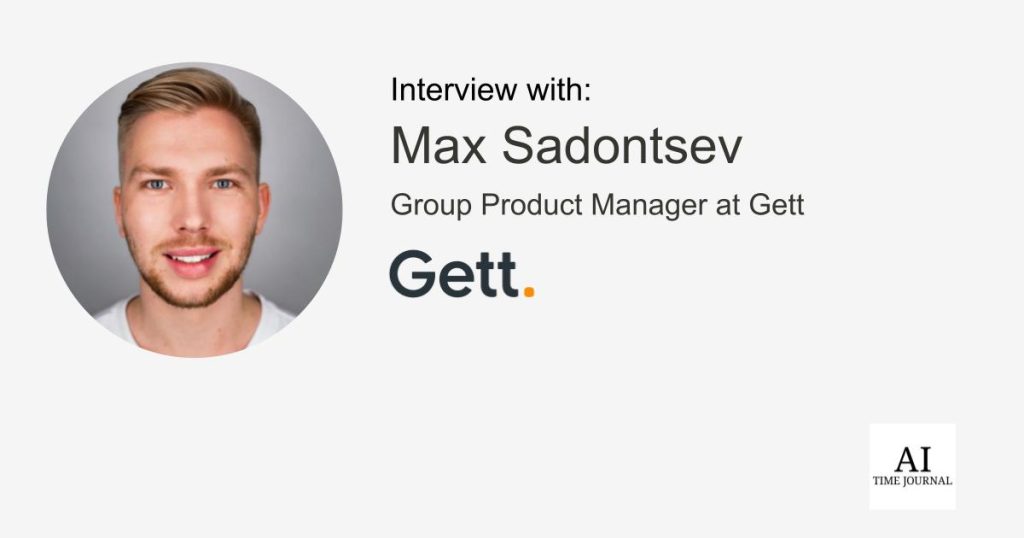
In the ever-evolving landscape of ride-hailing, the challenge of balancing immediate marketplace demands with long-term strategic goals is paramount. Max Sadontsev, the Group Product Manager at Gett, shares insights on navigating this complex terrain, emphasizing the importance of a clear vision. At Gett, machine learning (ML) and artificial intelligence (AI) have transformed operations, from efficient passenger-driver matchmaking to dynamic pricing during peak hours. By leveraging big data, Gett enhances customer experiences and boosts driver incomes. Looking ahead, Max envisions AI-driven innovations like advanced computer vision and generative AI revolutionizing transportation, making trips safer, cheaper, and faster. Despite regional regulatory challenges, Gett remains committed to regulatory compliance and innovation. This article delves into how Gett addresses diverse market needs, ensuring transparency and fairness, and explores the exciting potential of AI and ML in reshaping the ride-hailing industry.
Max, as the Group Product Manager at Gett, how do you balance the immediate needs of the marketplace with long-term strategic goals in such a fast-paced industry?
The most important part is to have the vision in place, first of all. It’s something that many PMs miss out when being buried under the extensive day to day work. Sit, relax, block some time, prepare it and communicate the vision to the stakeholders. Make sure everyone shares the vision.
Then, you can ensure that the immediate needs bring you to the long-term goal. And if not, maybe you made a misjudgement in your evaluations? A rule of thumb: make sure that about 80% of your tasks and roadmap matches with the vision and the rest can be dedicated to the quick wins outside of it.
Also, frankly speaking, the ride-hailing industry is in a somewhat of a stagnation point currently, with most of the companies being focused on profitability, rather than on growth.
Maybe, the next generation of AI tools will shake up the industry? Perhaps, it will be generative AI that revolutionises the transportation industry with the progressive self-driving cars.
Could you share a specific instance where machine learning significantly improved the efficiency of Gett’s operations?
Almost on every single step of a client interaction with the app. Think about the Gett app as a swiss knife compared to the traditional way of booking a taxi, which goes something like this. Calling a taxi station over the phone, providing your ride details manually. Waiting for a good half an hour for a driver to arrive. Being able to check where your driver is by calling the diver. Having to write a paper note to know how much you spent on taxi rides.
First, it was revolutionised by making every single step digital, via the app. However, everything worked by algorithms prepared by a developer: here’s how the address selection works, here’re the steps to find the best driver for you..
Machine Learning helps improve our algorithms by using Big Data and client/driver preferences to perform the best suggestions and the best matches. To make things even more accessible.
- You usually travel to your gym on Tuesday and Thursday mornings? Sure, we learned that and will suggest such a trip for you on these days;
- Not sure what’s the best curbside to be picked up from? No worries, we learned that through historical drivers behaviour;
- Who is the best driver to be assigned to your orders? We’ll get you covered by learning drivers preferences and make sure we offer first not just the closes driver but the closes driver to accept an order with parameters similar to yours;
- Are you afraid you won’t be able to take a ride during rush hour with all the cars being busy? The dynamic pricing tools will make sure that you will get a ride, whenever you need it. It is done by covering the extra fee over someone who might consider an alternative transportation option during rush hour.
Here are just some obvious examples of complex problems where ML delivers the best solutions to make our customers’ life easy.
How do you foresee AI and automation transforming the transportation industry over the next five years?
Sure, the current generation of the A.I., the Large Language Models are handy when it comes to supporting our customers and drivers on some issues, educating them in the form of a chat. With the abilities provided by the likes of Open AI, Amazon, IBM, Meta and others, any company can set up their own model, trained on tailored data that would relate to the specific knowledge. And not to the general knowledge of the society. And accurately answer some of the questions that consumers may have.
In addition, the LLMs can also be used to better interact with the data analytics and technical monitoring systems in a form of chat, rather than pure visuals or console logs.
I believe that the transportation market overall is not the biggest industry to be affected by these tools. Yet, the ride-hailing industry primarily solves problems in the different scopes, less related to the data input-output tools, human language interactions or context search.
However, advanced Computer vision and Generative A.I. alongside has the potential of finally transforming the way we all travel. As these technologies combined will finally bring autonomous driving everywhere. It would make your trips safer, cheaper and hopefully faster.
What unique challenges have you faced in managing a taxi booking platform that operates in both Israel and the UK, and how have you overcome them?
The main challenge is the unique specifics of each market, which means that our teams need to solve issues that are relevant only to the UK or Israel. That may frustrate the stakeholders from another country. So the first challenge is prioritising all of the needs in the correct order.
Next, I would say, the biggest marketplace challenge in Israel is the regulation that prohibits performing any dynamic price adjustments over the taxi metre. So we have to find creative solutions about how to engage enough drivers even during the toughest hours. For example, with non-monetary incentives. Also, somewhat unusual for the ride hailers. Recently we implemented an ML-powered solution that predicts how many passengers to expected to book a taxi from an airport in Tel-Aviv based on the arriving planes scheduled, as we recently won an airport tender and became an exclusive taxi service provider here.
And with the UK, for example, one of the interesting challenges is the dual market: you can book a licensed taxi, or a Black Cab. Or opt for a private hire service. We made a strategic decision that we would like to work in a traditional ride-hailing model only on the Black Cab market. And with the Private hire, we decided to partner with other companies, so we can offer the best of both worlds to our customers.
Overall, these markets still have many similarities in places and we always focus on building unified solutions, as much as possible.
In what ways has the integration of machine learning at Gett helped enhance the passenger and driver experience?
For customers:
- It takes 50% less time to book a taxi than before;
- You are 40% more likely to get a ride during peak hours;
- You’ve got 20% shorter driver search time, as we’ll find the most relevant driver for you right away;
For drivers: overall, we brought 30% higher incomes to the drivers.
Can you discuss the role of data-driven decision-making in your product management strategy at Gett?
I personally and our company follow the data-driven approach at our core. It helps avoid the bias in the decision making, as we would always assess the problem not by qualitative feedback from one customer but from a statistical asset of the metrics.
Likewise, we will set our priorities based on measurable ROIs of the initiatives and not by a subjective opinion of someone.
However, it’s very easy to abuse the data. Way before you can make data-driven decisions, you should first establish your metrics, build tracking tools (dashboards, reports), and define your KPIs. So you can always look at the big picture and relative changes.
Otherwise, you may, for example, see “this issue affects 1000 customers!”. Wow, sounds like a lot! We should solve it, don’t we? Well, what if it affected 1000 customers out of a million and worsened their experience only in 1% of the cases? Doesn’t sound as significant.
Lastly, we tend to always use the data during the new functionalities rollouts, A/B test the behaviours and make data-driven decisions on the impacts. And also always experiment with the configurations of the already rolled-out features – a continuous experimentation approach.
How do you ensure that the AI systems used at Gett are transparent and fair to both drivers and passengers?
Balance and fairness are at the core of the marketplace. Otherwise, it would become unbalanced and we would start to struggle to fulfil the rides. That would result in our business losing customers and drivers.
Naturally, every ML solution that we use at the marketplace is adjustable, so we can set up its biases, and goals that should be achieved. Over time, through experimentation and the models’ self-learning we constantly receive new insights from the data. We can always see its performance, set further KPIs to improve it and achieve even greater performance in the marketplace.
What innovations in machine learning are you most excited about, and how do you plan to incorporate them into Gett’s services?
Personally, the chatbots in particular help me a lot with my day-to-day productivity, as it just makes the data, the knowledge much more accessible. Unlike conventional search engines, bots help me find the right answers much faster.
I’m sure that very soon, with deeper integrations of the advanced ML models into the OS of our devices and services that we use, both the personal and professional routines will be optimised quite significantly.
As for the enterprises of different kinds in general, I believe the biggest revolution would be about advanced analysis of Big Data. So the companies will be able to make data-driven decisions much more efficiently.
And, well, for the software companies, it might be the generative AI capable of writing the code of any sorts, supervised by human developers. This way, some new apps of a new kind that we couldn’t even imagine might be born!
As Gett, we are entirely open to the new technologies and would be keen to integrate any of those to our internal processes or consumer-facing products.
We are already experimenting with the LLM models internally. As soon as the new solutions arrive, we will see how we can adopt them. We have been experimenting with the autonomous cars idea together with the VW Group ever since 2017.
How does Gett address the varying regulatory environments and customer expectations in different regions it operates in?
Gett always complies with the regulatory requirements, being a licensed taxi service provider. However, the beauty in this situation is that most regulators are open for the feedback that we as the company can translate from our customers and drivers.
For example, we are being vocal currently about the shortage of the new Black Cab drivers in the UK that affects our service reliability to the customers directly. And working with the TFL (Transport for London) on creating new onboarding tools for drivers, including our own onboarding centre.
Could you elaborate on how taxi hailing machine learning algorithms match passengers with drivers and the key factors that influence this process?
The matchmaking process in itself is a complex algorithm that consists of both ML-driven and regular flows.
Unfortunately, I’m unable to share all of the Gett’s secret sauce but let me share just one example:
All drivers are set in different conditions during the matchmaking process:
- Every driver has a unique distance to drive towards the pickup location;
- Some are still busy with completing another ride nearby;
- Some drivers have just completed a short ride that wasn’t that profitable. And the others just did a long journey from the airport ;
- Some drivers really love to operate in the area of the ride destination and others don’t;
- Some drivers enjoy cash rides and others hate it.
We train an ML model on a set of the features, including the ones I mentioned above, assign a weight (importance) of each. And during the matchmaking process, taking just milliseconds, the ML model predicts the likelihood of each possible driver candidate to accept this order and helps us rank drivers correctly in the order of priority.