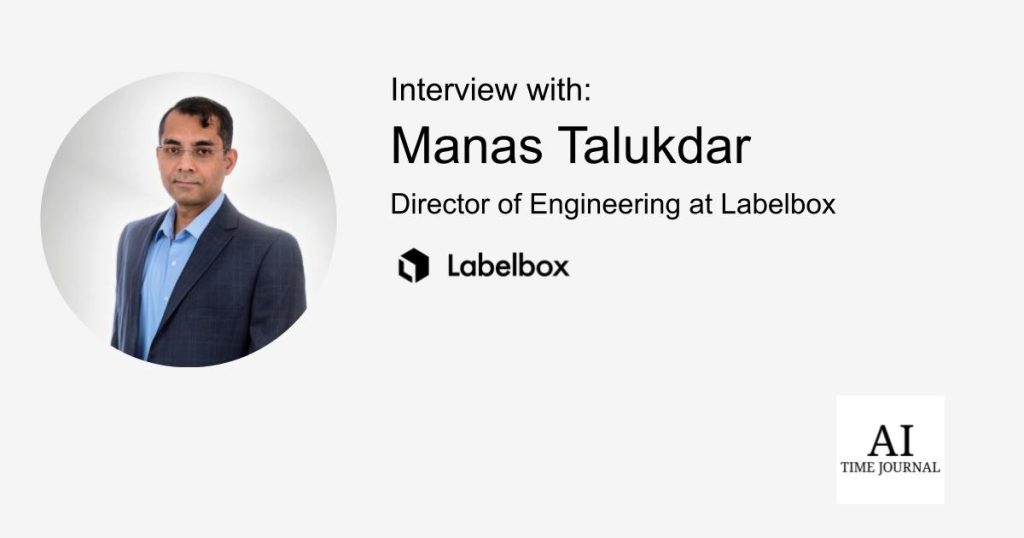
Manas Talukdar, the Director of Engineering at Labelbox, has an extensive career in artificial intelligence and data infrastructure. His journey began with a pivotal project involving the development of a cloud-native data platform prototype, which significantly shaped his understanding of scalable and reliable data systems. This foundational experience propelled him into leading roles where he built AI platforms for major enterprises, tackling challenges such as predicting rust rates in oil pipelines using AI. At Labelbox, Manas is at the forefront of innovation, spearheading projects that enhance multi-modal large language models, directly impacting AI development across consumer and enterprise spaces. His balanced approach to innovation and reliability ensures the creation of robust systems capable of critical decision-making in real-world settings. Manas’s insights into the evolving landscape of AI and his leadership in developing cutting-edge technologies make him a significant figure in the AI and data science community.
Your journey in the field of artificial intelligence and data infrastructure has been remarkable. Could you share some pivotal moments or challenges that significantly shaped your career?
Many years back I got the opportunity to work on a research project to help build out a prototype for a cloud-native data platform. This was a pivotal moment in my career as it allowed me to work on a cutting-edge technology stack and learn about the challenges of building large-scale data infrastructure systems. Subsequently I got the opportunity to build and lead a team taking this prototype to production, as well as implement support for data science use cases in the data platform. This experience helped me understand the importance of building scalable and reliable systems to support data science workflows, and has been instrumental in shaping my career in the field of AI and data infrastructure.
Later on I worked for the leading enterprise AI company and helped build an AI platform. During the early days of that stint I got the opportunity to learn of a use case where a customer in the energy sector wanted to use AI to predict rust rates in their oil pipelines by training and infererencing on a variety of data including drone based pictures of their pipelines. This was a key moment for me as it helped me understand the importance of building AI systems that are reliable and can be trusted to make critical decisions in real-world settings across different industries.
These and other similar experiences have played important roles in my over decade and a half long career in the field of AI and data infrastructure.
As the Director of Engineering at Labelbox, what are some innovative projects or initiatives you are currently spearheading that you believe will have a major impact on the industry?
Right now there is an arms race going on to build increasingly powerful multi-modal large language models. At Labelbox we are shipping capabilities in our AI platform that enable AI labs to develop these powerful multi-modal LLMs. I am really excited about this work as it directly influences the cutting edge of AI development and the tremendous impact these AI models will have on both the consumer as well as enterprise space.
Given your extensive experience in developing products for mission-critical sectors, how do you approach the balance between innovation and reliability in your engineering practices?
I give equal importance to both innovation and reliability in my engineering practices. I believe that innovation is key to staying ahead of the competition and delivering value to customers, while reliability is key to building trust with customers and ensuring that the products we build can be used in mission-critical settings. I approach this balance by ensuring that while we are keeping up with the cutting-edge research and constantly innovating, we are at the same time adequately managing technical debt and are building robust systems that can be trusted to make critical decisions in real-world settings.
In your opinion, what are the most significant trends in Enterprise AI today, and how should businesses prepare to leverage these advancements effectively?
Currently Generative AI is a hot topic in the AI space and this is reflecting in the enterprise AI world as well. Businesses are increasingly investing in leveraging generative AI models to generate high-quality content across different modalities. These models have the potential to revolutionize the way businesses create content and interact with customers. Companies are looking to use Gen AI to get quick, actionable insights from massive amounts of data across different data sources and types.
Businesses should prepare to leverage these advancements by investing in the right talent and infrastructure to utilize these generative AI models at scale. They should focus on building robust data pipelines to support the training and inferencing of these models, as well as invest in the right tools and platforms to monitor and manage these models in production.
You have been recognized through multiple awards and have served as a judge for prestigious industry awards. What do you consider the key criteria for excellence in AI and data infrastructure projects?
Key criteria for excellence in AI and data infrastructure projects include the ability to scale to handle large volumes of data, the ability to integrate with other systems and tools, the ability to support the relevant data science use cases, and the ability to deliver high-quality results in a timely manner. Projects that excel in these areas are more likely to be successful and have a positive impact on the business. It is also important to plan out these complex projects in a way that is agile and iterative, so that the team can quickly adapt to changing requirements and incrementally deliver value to the business.
How do you envision the future of work evolving with the increasing integration of AI and automation in business processes? What skills do you believe will be most crucial for professionals to thrive in this environment?
AI will continue to play a key role in automating routine tasks and augmenting human decision-making in the workplace. Professionals who are involved in developing AI will need to have a strong understanding of the underlying algorithms and models, as well as the ability to work with large volumes of data and build scalable systems. Those that are involved in using AI will need to have a strong understanding of how AI works, how to leverage and integrate with machine learning models and how to interpret the results, as well as the ability to work with AI systems in a way that is ethical and responsible. In addition, professionals will need to have strong communication and collaboration skills, as AI will require cross-functional teams to work together to develop and deploy AI systems. Domain knowledge is also important, as AI systems are often developed to solve specific problems in specific industries.
Your role involves leading multiple teams in developing large-scale systems. What are some leadership strategies or principles that you find most effective in fostering innovation and collaboration within your teams?
I generally follow the following leadership strategies and principles to foster innovation and collaboration within my teams:
- Encourage open communication and collaboration. I aim to create an environment where team members feel comfortable sharing their ideas and working together to solve problems. This includes having the psychological safety to speak up, share their thoughts and ideas, and even disagree with their peers and leaders.
- Foster a culture of continuous learning and improvement. I encourage my team members to keep up with the latest research in the field of AI and data infrastructure both in industry and academia and look for ways to incorporate them in our work and roadmap. I also encourage them to utilize any company benefit for learning and development to take courses, attend conferences, and participate in workshops.
- Provide clear goals and objectives. I work with my teams to define clear goals and objectives for each project, and ensure that everyone understands their role and responsibilities in achieving these goals. Goals and objectives are also important and relevant for career progression plans.
- Balance cross-pollination with focus and specialization. I encourage my team members to work across different projects and teams to gain exposure to different technologies and domains, while also allowing them to specialize in areas that they are passionate about and excel in.
With AI continuing to impact both business and academia, what do you think are the most critical areas where AI will drive significant change in the next decade?
AI will continue to affect every aspect of our lives in the next decade. Some of the most critical areas where AI will drive significant change include healthcare, finance, transportation, and education. In healthcare, AI will help doctors diagnose diseases more accurately and quickly, and help researchers develop new treatments and cures for diseases. In finance, AI will help companies make better investment decisions and manage risk more effectively. In transportation, AI will help companies develop autonomous vehicles and improve the safety and efficiency of transportation systems. In education, AI will help teachers personalize learning for students and improve the quality of education for all. We are also seeing AI being used in climate change, energy, and even in astrophysics. There are in fact custom LLMs being developed for domain specific tasks and the results are very positive. With developments in quantum computing AI will affect human society and development in ways some of which we probably cannot yet fully imagine. The possibilities are endless and the impact will be profound.
As an advisor to startups in the AI and Data space, what common challenges do you see these emerging companies facing, and what advice do you offer to help them succeed?
One of the biggest challenges currently facing emerging startups is the change in the capital market. The capital market is currently in a state of flux, with investors becoming more cautious and selective in their investments. This has made it difficult for startups to raise the necessary funding to grow and scale their businesses. My advice to these startups is to focus on building a strong product and team, and to be patient and persistent in their efforts to secure funding. In a way this challenge is actually good for the industry. Founders are now pivoting to focus on building a good product and think about product market fit and revenue generation as opposed to being able to raise large amounts of money without any discernible revenue stream. It is important for startups to focus on building a strong customer base and generating revenue, as this will help them attract investors and grow their businesses. I also work with them to review their product and provide ideas for improvements from both engineering and product aspects. I help them to think about their engineering organization and how to structure it for success. I encourage them to think about their possible target segment in the market and how to position themselves to be successful relative to others in the space.
The development of powerful language models (LLMs) relies heavily on data. How do you see the role of data evolving in the context of AI, and what are the key considerations for ensuring high-quality data in AI projects?
Data curation and quality are key to the success of AI projects. As the field of AI continues to evolve, the role of data will become even more important. It is crucial to ensure that the data used to train and infer these models is of high quality and representative of the real-world scenarios that the models will be used in. This requires investing in data quality tools and processes, as well as building robust data pipelines to support the training and inferencing of these models. With the increasing number of domain specific LLMs there will also be a need for high-quality annotated data to train these models. This will require investing in data annotation tools and processes, as well as building a strong and specialized data labeling team to ensure that the data is labeled accurately and consistently. Some cutting-edge work is also looking into reward-model-as-judge for evaluating the quality of the data including LLM responses. This will be an interesting area to watch in the coming years.