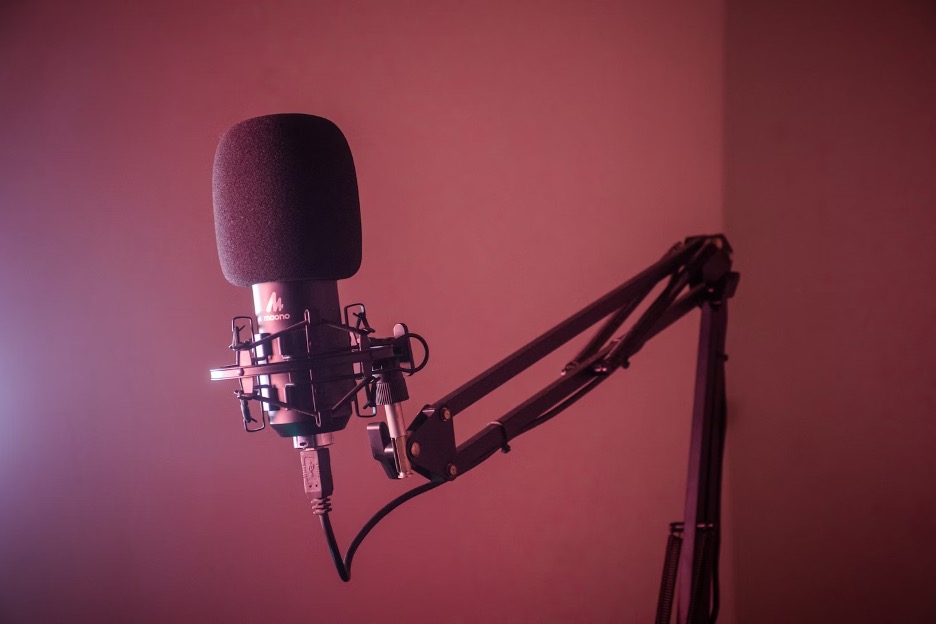
Speech recognition technology is now a vital component of our digital world, driving virtual assistants, transcription services, and more. The demand for accurate and efficient speech-to-text systems continues to rise, and automation in AI has become essential to meeting this need. By leveraging automation, these systems can achieve higher performance, greater reliability, and scalability.
This article explores the role of automation in enhancing speech recognition and provides practical steps to implement it for better results.
In 2024, the number of voice assistant users is projected to reach 8.4 billion, doubling from 4.2 billion in 2020. This rapid growth emphasizes the increasing demand for automatic speech recognition systems that can deliver higher accuracy and faster responses. Automation in AI is critical in meeting these demands, enabling more efficient and effective speech recognition.
Automation’s Impact on AI-Powered Speech Recognition
Automation in AI has revolutionized speech recognition technology. By automating various processes, AI can handle vast amounts of data and improve the accuracy of voice recognition systems. Here are key areas where automation plays a vital role:
- Data annotation. Automation streamlines the data annotation process, allowing for the rapid labeling of large datasets. This is essential for training AI models in automatic speech recognition systems, ensuring they can handle diverse speech patterns and accents.
- Continuous learning. Automated systems support continuous learning, where models are updated with new data regularly. This process ensures that speech recognition systems stay current and accurate, adapting to new languages, dialects, and speech patterns without manual intervention.
- Error reduction. Automation reduces human errors in data processing. By minimizing these errors, AI-powered speech recognition systems achieve higher accuracy and reliability. This improvement is crucial for applications where precision is paramount, such as in healthcare or legal transcription services.
The integration of automation in AI-powered speech recognition systems enables the handling of complex tasks with greater efficiency. As automation continues to evolve, its role in enhancing these systems becomes more significant. The ability to process and analyze large datasets automatically ensures that automatic speech recognition systems remain robust and responsive to the ever-growing demand.
How to achieve Better Speech Recognition Performance?
Achieving better performance in speech-to-text systems requires a combination of strategic approaches and technological enhancements. The goal is to improve accuracy, reduce processing time, and handle diverse speech patterns more effectively. Here’s what you can do to make these enhancements a reality.
1. Use High-Quality Data for Training
The quality of the data used to train AI models is the foundation of any successful speech-to-text system. Poor-quality audio data leads to poor model performance, regardless of the sophistication of the AI algorithms. Therefore, focus on:
- Collecting clear and diverse audio samples from various environments.
- Ensuring that your training data includes different accents, dialects, and speech speeds.
- Regularly updating your datasets to reflect changes in language usage and emerging speech patterns.
2. Implement Automated Data Annotation
Manual data annotation is time-consuming and prone to errors. Automating this process speeds up model training and enhances accuracy. Automated data annotation tools can label large datasets more consistently, improving the quality of the data fed into your models. This leads to better performance in transcribing audio-to-text tasks.
3. Optimize Model Architectures
Choosing the right model architecture is key to improving performance. Some models are better suited for handling specific tasks like noisy environments or recognizing unique accents. When optimizing model architectures:
- Test different models and select the one that offers the best balance between accuracy and processing speed.
- Consider models that can handle real-time transcribed audio-to-text tasks, especially for applications requiring instant feedback.
- Continuously monitor and refine model performance based on new data.
4. Leverage Continuous Learning
AI models for speech-to-text systems should never remain static. Continuous learning allows models to adapt to new speech patterns, languages, and environments. Regularly updating models with new data ensures they remain accurate and effective over time.
5. Monitor and Measure Performance Regularly
Regular monitoring and performance measurement are critical for maintaining and improving speech-to-text systems. By keeping a close eye on how well the system performs under different conditions, you can identify areas for improvement.
Steps to Implement Automation for Enhanced Speech Recognition
To implement automation for enhanced voice to text systems, follow these steps. Each step helps streamline the process, making your audio transcription more efficient and accurate.
1. Choose the right automation tools
Start by selecting the tools that align with your specific needs. If your transcription involves video or multimedia content, consider tools that combine audio transcription with computer vision technology. For example, in video recordings, computer vision can help identify and analyze visual cues, such as lip movements or contextual visuals.
2. Prepare and organize your data
Before automation can be effective, organize your data. Ensure that your audio and video files are clear, properly labeled, and representative of the various speech patterns you want to recognize. This preparation helps the automation tools work more efficiently and improves the final output of your voice-to-text system.
3. Automate data annotation
Automate the data annotation process to speed up the training of your AI models. Automation reduces manual errors and allows for consistent labeling across large datasets. With accurate annotations, your models will better recognize and transcribe diverse speech patterns.
4. Train and optimize your AI models
Once your data is annotated, use it to train your AI models. Optimize the models by testing them with different datasets to identify the most effective configuration. Focus on models that offer the best balance between speed and accuracy, especially for real-time audio transcription tasks.
5. Implement continuous learning
Set up a system for continuous learning to keep your AI models up-to-date. Regularly update the models with new data and user feedback to ensure they adapt to changing language patterns and environments. This step keeps your voice-to-text system performing at its best over time.
Final Thoughts
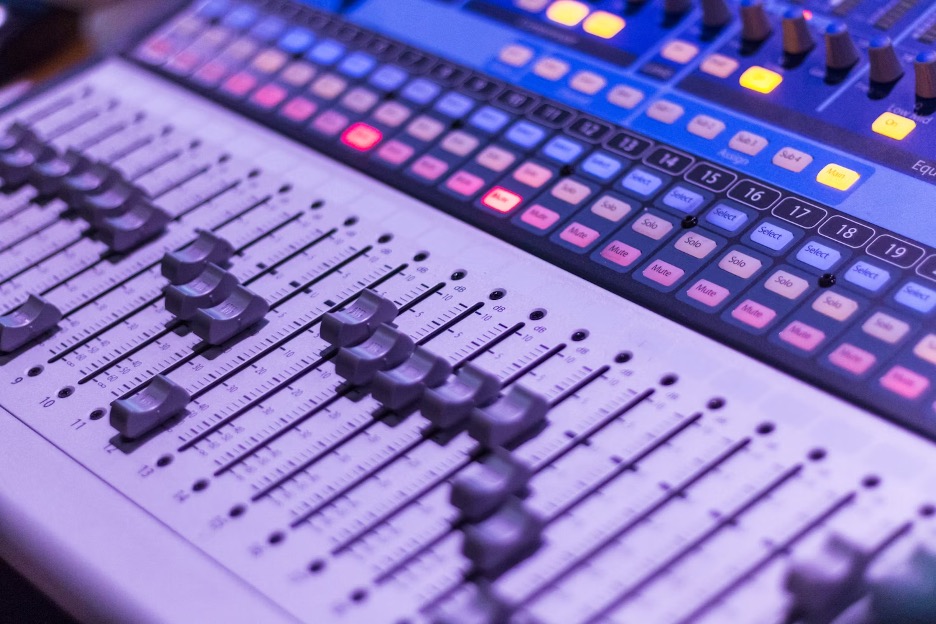
Automation in AI is a powerful tool for advancing speech-to-text systems. By focusing on high-quality data, optimizing model architectures, and implementing continuous learning, these systems can achieve better efficiency. The steps outlined in this article provide a clear path to harnessing automation for superior speech recognition performance. As the demand for reliable and scalable audio transcription grows, adopting these strategies will be key to staying ahead in this rapidly evolving field.