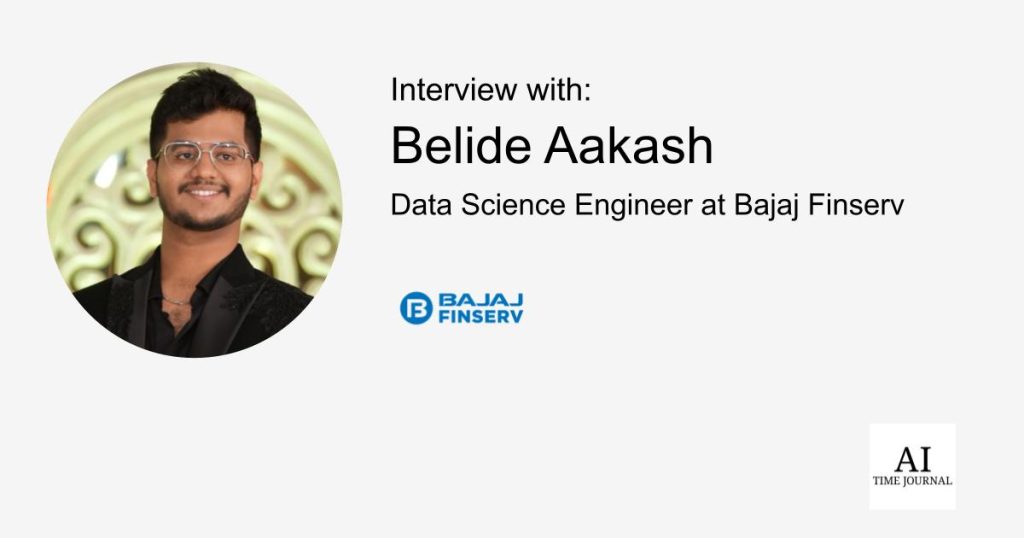
The intersection of AI, automation, and data science is transforming industries across the globe. One sector experiencing significant upheaval is financial services, where innovative technologies are reshaping customer experiences and operational efficiencies. In this insightful interview, we welcome Belide Aakash, a data science professional at Bajaj Finserv, to explore how AI and automation are revolutionizing the non-banking financial company (NBFC) sector. We also discuss the future of work in an AI-enhanced world, offering valuable advice for aspiring data scientists and insights into the ethical implications of these powerful technologies. From reducing loan approval times dramatically to implementing cutting-edge AI-driven solutions, discover the challenges and triumphs faced along the way.
Can you walk us through your journey into data science and what led you to your current role at Bajaj Finserv?
Since college, I have always been fascinated by data. I had done multiple projects in the field during my internships and eventually cracked the on-campus placement offer for a data science role at Bajaj Finserv.
How do you see AI and automation transforming the financial services industry, particularly in non-banking financial companies (NBFCs) like Bajaj Finserv?
NBFCs focus on the business domain and data is the core part of their journey. Starting from onboarding a customer, till their loan repayments are done on time, technical automation plays a very important role. There was a period when the customer had to wait for around 3 days to get their loan application approved. AI models built on clean data and automation have brought it down to almost 3 minutes. That is like 1440 times faster! Working on scale is one of the important factors that leads to such mega changes.
What are some of the most innovative AI-driven solutions you have implemented or worked on at Bajaj Finserv?
I have worked on various AI-based propensity models, risk scorecards, etc which have helped the business stakeholders. Unfortunately, I cannot share further details due to NDA.
In your experience, what are the biggest challenges businesses face when integrating AI and automation into their operations?
I believe improper planning and communication is one of the biggest challenges during such processes. It is important to plan out the timeline which should be extrapolated based on an initial POC. Furthermore, we need to plan out and check for the budget requirements for all the infrastructure required for the projects well before and also have sufficient planning for disaster-recovery as well. Once the project is in operation, having meetings that would lead to tangible and transparent outcomes would result in a better output. The quality might deteriorate due to improper communication between multiple teams.
How do you envision the future of work evolving with the increasing presence of AI and automation in various industries?
AI has always been in almost every industry but only in terms of AI and ML models. But for the past two years, Generative AI has changed its meaning and even a person with no coding background can utilize AI to its fullest potential. No code platforms like Make, Zapier, and Bardeen along with APIs from OpenAI, Gemini, etc have opened up many potential use cases. Someone from a technical background can further boost it due to its flexibility. I believe AI and automation would revolutionize many content creation jobs, logistics, sales, marketing, customer services, and many other industries that require a human-like decision-making process in the workflow.
What advice would you give to young professionals and students aspiring to build a career in data science and AI?
Back in my college days, we never had access to AI tools such as ChatGPT, Gemini or Claude. Today, it’s completely different. Irrespective of whatever field people are in, I would suggest everyone focus on the following things:
- Using AI tools
- Prompt Engineering
- AI Agents and workflows
These will be the most demanded fields and skills that people will need in the future. A person who knows how to use AI tools properly with decent skills would be 10 times more preferable over a person who doesn’t know how to use AI tools but has very good skills. This is because the first person would be able to do a task within 2 hours while the same person might spend more than 6 hours.
Coming to Data Science, I would suggest either going deep into topics like Data Engineering, Data Management, ML, and AI or having a partial idea in a few of these along with some ideas on backend technologies (especially APIs) and DevOps. People are looking for all-rounders. It’s because they will know how to integrate multiple tools.
Could you discuss the role of data engineering in supporting and enhancing AI capabilities within financial services?
Financial services heavily depend on CLEAN and USABLE data. Irregularities in data and improper database schemas can lead to huge discrepancies. Furthermore, there are a lot of regulations that have to be followed based on government restrictions. Data engineers come to solve all such problems. From maintaining data in the datalake to building scalable pipelines to extracting valuable insights from data, it is all done by them.
How do you think AI agents will shape the future of customer interactions and service delivery in fintech?
Having normal AI agents based on OpenAI or Gemini models wouldn’t be enough in the fintech industry. It is essential to have AI agents built upon internal data for personalized interactions. Doing this will enable the model to have access to understand customer behaviour and based on that, the replies would differ. If a person has defaulted many times, the model would treat them strictly while it would be a little lenient on a good customer.
Beyond your professional expertise, what are your thoughts on the ethical implications of AI and its potential impact on society?
With the emerging trends in AI, many people are finding ways to bypass using prompt injections, and multi-step examples to break the models and get what is required. It is important to perform prompt sanitization while releasing solutions in production. Furthermore, certain models are biased towards certain topics like race, gender, etc which is a breach. Such biases have to be managed before releasing them to the public. One such scenario involves Google’s text-to-image model producing racial images that had a serious backlash from the public.